The Promise and Pitfalls of AI Document Interpretation
- Staff Desk
- Oct 28, 2024
- 10 min read
Navigating the Accuracy and Limitations of AI-Powered Document Processing
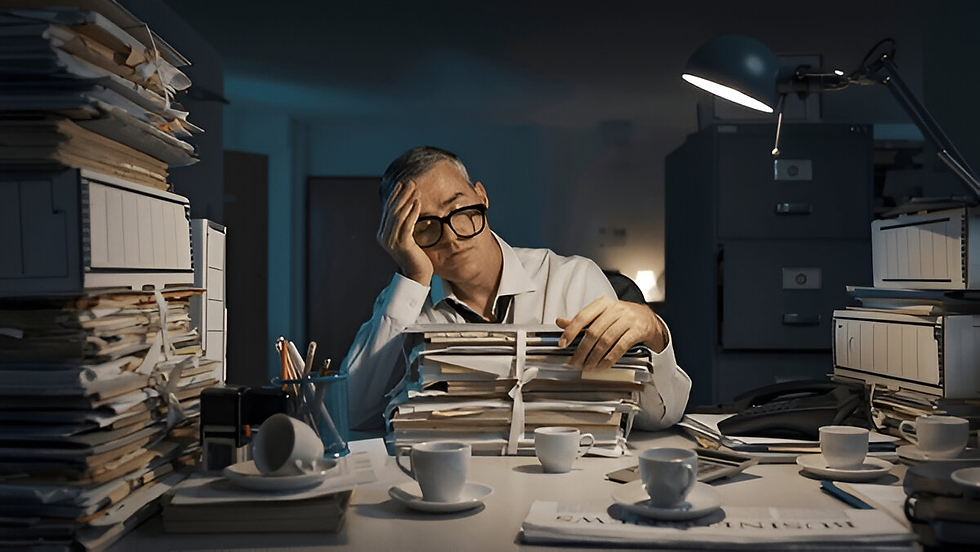
AI document interpretation has gained significant attention in recent years as businesses and individuals seek to automate and enhance their document processing tasks. The accuracy of AI document interpretation can vary greatly depending on several factors, including the quality of the input data, the specific technology used, and the complexity of the documents being analyzed.
As AI technologies like natural language processing and machine learning continue to evolve, they provide impressive capabilities for understanding and extracting information from various document types. However, limitations still exist, particularly with nuanced language and contextual understanding, which can impact accuracy.
This article explores the advancements in AI document interpretation, the challenges it faces, and the real-world implications of its accuracy. Readers will gain insight into what can be expected from this technology and how it can be effectively utilized.
History and Evolution of AI in Document Interpretation
The journey of AI in document interpretation has seen significant advancements. From its rudimentary beginnings to the sophisticated systems used today, several key developments have shaped this field.
Milestones in AI Development
The origins of AI date back to the early 20th century. In 1919, the conversation around intelligence began to evolve with Alan Turing's foundational ideas emerging a few decades later. Notable milestones include the introduction of natural language processing (NLP) in the 1950s, which enabled computers to understand human language.
In the 1980s, expert systems gained popularity, utilizing predefined rules to assist in decision-making. These early systems provided basic document interpretation but were limited in scope. The 1990s saw the first wave of machine learning techniques applied to text analysis, setting the stage for more advanced approaches.
From Rule-Based Systems to Machine Learning
Rule-based systems dominated the early years of AI document interpretation. They operated on a fixed set of algorithms and rules, which restricted their adaptability. This approach worked well for structured documents but faltered with unstructured data common in natural language.
The advent of machine learning shifted the paradigm. Algorithms learned from data, allowing for improvements in accuracy over time. This transition began in the late 1990s and continued into the 2000s, leading to better performance in parsing and understanding documents. Machine learning facilitated the development of algorithms that could account for context, making AI systems more flexible and responsive.
The Role of Neural Networks and Deep Learning
Neural networks revolutionized document interpretation in the 2010s. With the rise of deep learning, algorithms became capable of processing vast amounts of unstructured data more effectively. Models like recurrent neural networks (RNNs) and convolutional neural networks (CNNs) enhanced the ability to analyze complex documents.
Generative AI, a subset of deep learning, has also impacted this field. It allows for the generation of text and interpretation of documents in innovative ways. While these advancements continue, concerns around security and ethical implications have emerged, particularly as AI models become more pervasive in sensitive areas like healthcare and finance.
The evolution from early AI systems to modern deep learning techniques reflects a rapid advancement in capabilities. Innovations continue to push the boundaries of how AI interprets documents, shaping its future applications.
Fundamentals of AI Document Interpretation
AI document interpretation relies on advanced technologies and quality data. Understanding how these elements interact is vital for establishing accuracy in processing and analyzing documentation.
Understanding Natural Language Processing (NLP)
Natural Language Processing (NLP) is essential for AI's ability to interpret text. It enables machines to understand, analyze, and generate human language. NLP techniques include tokenization, part-of-speech tagging, and named entity recognition.
AI tools leverage NLP to assess context, intent, and sentiment. For instance, Composer AI utilizes NLP capabilities for effective document drafting and editing. This technology not only enhances clarity but also improves the overall coherence of documents.
Accurate interpretation hinges on sophisticated models trained to manage linguistic complexities. As these models evolve, they enhance the ability to interpret diverse document types, from legal texts to creative writing.
The Importance of Data Quality and Volume
Data plays a crucial role in the performance of AI interpreters. Quality data involves well-structured, comprehensive, and relevant information tailored to specific tasks. The more representative the dataset, the better an AI can learn and generalize.
Volume is equally important. Large datasets increase the chances for AI systems to encounter and learn from various document structures and formats. This exposure helps to create more robust models that can handle real-world complexities.
Generative AI faces challenges when dealing with biased, incomplete, or noisy data. Such limitations can lead to inaccuracies in document interpretation, ultimately affecting the reliability of the AI’s outputs.
Challenges and Limitations
Despite advancements, AI document interpretation faces notable challenges. Ambiguities in language, idiomatic expressions, and cultural contexts can hinder accurate understanding.
Moreover, generative AI often struggles with context retention over long documents. It may misinterpret or overlook crucial information as it processes text sequentially.
Another significant limitation pertains to the quality of input data. Inaccurate or biased datasets can lead to poor interpretations and decision-making. Addressing these challenges is critical for improving accuracy, making ongoing research and development essential for enhancing AI’s capabilities in document handling.
AI and Document Review
AI technology plays a significant role in document review across various fields, particularly in legal and academic contexts. Its ability to analyze large volumes of text efficiently has transformed how professionals handle document assessment.
AI in Legal and Regulatory Compliance
In the legal domain, AI tools are employed for tasks such as contract review, due diligence, and e-discovery. They can swiftly scan documents, highlighting key clauses and flagging potential issues.
Key capabilities include:
Document classification: Sorting documents based on relevance.
Keyword identification: Finding critical terms that impact case outcomes.
Risk assessment: Evaluating language that may pose compliance issues.
Examples of AI tools used in this field include ContractPodAI and Kira Systems. These technologies improve accuracy and reduce the time lawyers spend on routine reviews.
AI in Academic and Research Document Review
AI's influence extends to academic document review, where tools assist with literature reviews and source identification. These systems can evaluate thousands of scholarly articles to find relevant research.
Benefits include:
Citation tracking: Identifying sources cited by other papers.
Plagiarism detection: Ensuring originality of research submissions.
Content summarization: Providing concise overviews of complex studies.
Platforms like EndNote and Mendeley leverage AI for these purposes, enhancing the efficiency of researchers in gathering and reviewing literature. The ability to find credible sources quickly supports accurate referencing and strengthens academic work.
Accuracy and Efficiency in AI Interpretation
AI's ability to accurately interpret documents is critical for enhancing efficiency in various sectors. This discussion covers the comparative accuracy of AI versus human interpretation, how AI improves over time, and the metrics used to assess AI's accuracy.
Comparative Accuracy Between AI and Humans
When comparing AI and human interpretation, AI systems have shown impressive performance in tasks such as text analysis and data extraction. Certain platforms, like Google's Cloud Natural Language and IBM's Watson, demonstrate accuracy that often surpasses human capability in specific contexts, particularly in handling large volumes of data quickly.
Nevertheless, human context understanding can sometimes lead to more nuanced interpretations. While AI excels in consistency, it may struggle with ambiguous language. Therefore, in complex scenarios, such as legal documents, collaboration between AI and human experts is advised for optimal results.
Improving AI Interpretation Over Time
AI interpretation accuracy is not static; it continuously improves through machine learning and user feedback. Platforms like OpenAI and Microsoft Azure incorporate user inputs to refine their algorithms, leading to better outcomes with each iteration.
Furthermore, the addition of training data enhances AI’s contextual awareness. As diverse datasets are introduced, AI becomes increasingly capable of understanding various nuances in language and context. Regular updates and advancements in natural language processing techniques contribute to this ongoing improvement.
Metrics for Measuring AI Interpretation Accuracy
To evaluate the accuracy of AI document interpretation, specific metrics are employed, including precision, recall, and F1 score.
Precision measures the ratio of relevant instances among the retrieved ones.
Recall assesses the proportion of relevant instances that have been retrieved over the total relevant instances.
F1 Score balances precision and recall into a single metric.
Many organizations use these metrics to choose the most accurate AI platform for their needs. Accuracy testing can also be domain-specific, ensuring the selected AI tools perform well in the required context, such as storyboarding for video concepts or other specialized tasks.
AI Technologies and Their Applications
AI technologies serve various purposes, particularly in content generation and enhancing user experience. By leveraging sophisticated algorithms, these technologies provide significant advancements in how information is processed and presented.
Generative AI and Content Creation
Generative AI plays a pivotal role in content creation by generating text, images, and videos based on specific inputs. This process involves algorithms that learn from vast datasets, allowing for the production of tailored content.
For instance, custom number settings can be employed with basic AI tools to refine outputs, ensuring relevance to specific user needs. Industries utilizing generative AI can streamline marketing efforts by automating ad copy or producing creative visuals.
In video generation, the training of AI models on diverse video datasets allows for enhanced video editing capabilities. It can automate subtitling or generate promotional clips, thus increasing production efficiency while reducing manual effort.
AI for Optimizing User Experience (UX)
AI significantly influences UX design through personalized experiences. By analyzing user behavior and preferences, AI can create more engaging interfaces and suggest relevant content.
Tools like chatbots utilize natural language processing to improve customer interactions, offering instant support. This technology not only meets immediate needs but also gathers data for continuous UX improvement.
Furthermore, AI helps in A/B testing, allowing designers to identify which elements work best through real-time data analysis. This iterative process optimizes engagement and conversion rates, leading to more effective digital products.
Implementing AI Within Organizational Workflows
Integrating AI into organizational workflows requires careful consideration of specific use cases and the compatibility of AI tools with existing systems. Ensuring that AI technologies align with business processes enhances their effectiveness and boosts productivity.
Use Cases and Testing AI Systems
Identifying use cases for AI is crucial. Organizations should focus on areas where document interpretation can bring significant benefits, such as data extraction, contract analysis, and compliance monitoring.
Testing AI systems before full implementation involves creating pilot programs. Teams can deploy AI tools in specific departments to evaluate performance. Metrics to consider include accuracy rates, user satisfaction, and processing speed. Gathering employee feedback early can aid in refining AI capabilities. Regularly assessing these systems ensures they evolve with organizational needs.
Integrating AI with Existing Software and Platforms
Successful integration of AI tools demands a thorough analysis of current software and workflows. Organizations must assess compatibility with existing systems such as CRM, ERP, or document management solutions.
Collaboration with IT teams is essential for seamless integration. Options include API connectors or custom-built interfaces tailored to specific needs. Training employees to use new AI features effectively is critical for adoption.
An organized approach to integration will enhance user experience and optimize business processes. A structured rollout plan can minimize disruptions while maximizing the impact of AI technologies.
Ethical Considerations of AI Document Interpretation
The ethical implications of AI document interpretation encompass various crucial aspects such as bias, privacy, and the evolving job landscape. Addressing these concerns is essential for ensuring responsible AI deployment in real-world applications.
Bias and Fairness in AI
Bias in AI systems can result from the data used for training or the algorithms applied. When document interpretation tools are trained on biased datasets, they may produce skewed outcomes, affecting fairness in decision-making processes.
Implementing fairness measures during AI product development serves to identify and mitigate these biases. Techniques such as adversarial debiasing can help in creating more equitable AI systems. Additionally, continuous auditing is necessary to assess AI compliance with standards like ISO 9001, focusing on quality management and operational efficiency.
Privacy and Data Security Concerns
AI document interpretation often involves processing sensitive information. This raises significant privacy and data security challenges. Ensuring that AI systems comply with regulations, such as GDPR, is vital to protect user data.
Organizations must establish robust data protection measures. Techniques like data anonymization and encryption can safeguard personal information during processing. Furthermore, transparent data handling policies can build trust among users regarding how their data is utilized and stored.
The Future of Work with AI Automation
AI automation in document interpretation will inevitably transform the workplace. While it can enhance productivity, there are concerns about job displacement as tasks become automated.
Workers may need to adapt by acquiring new skills relevant to managing and collaborating with AI technologies. Emphasizing lifelong learning is crucial for employees to remain competitive. The integration of AI also offers opportunities for improved auditing practices, ensuring compliance while enhancing operational procedures.
Emerging Trends in AI and Document Analysis
Recent developments in artificial intelligence are significantly enhancing document interpretation and analysis capabilities. These advancements are primarily driven by improvements in machine learning and predictive analytics, which provide valuable insights for decision-making processes.
Advancements in Machine Learning Models
Machine learning models are evolving rapidly, enabling more accurate document interpretation. New architectures, such as transformer models, have improved natural language processing capabilities. These models can understand context, sentiment, and intent more effectively.
For example, AI systems can analyze resumes and other recruitment documents with a much higher degree of precision. This allows organizations to streamline their hiring processes and make data-driven decisions.
Additionally, the integration of AI with social media platforms is reshaping recruitment strategies. Companies can leverage AI to identify potential candidates by analyzing their online activity, skills, and experiences reflected in their profiles.
Predictive Analysis and Decision Making
Predictive analytics plays a crucial role in enhancing document analysis. AI can analyze historical data to forecast trends and outcomes. For instance, chatbots can assist in cost analysis by evaluating responses and predicting future requirements based on existing patterns.
Organizations are now using AI tools to perform cost assessments more efficiently. By processing large volumes of documents and extracting relevant data, AI helps in optimizing budgets and improving financial planning.
Moreover, as these AI-driven systems learn from ongoing interactions, they continually refine their accuracy in predictive analysis. This adaptability leads to more informed decisions and better resource allocation in various sectors.
Practical Tips for Using AI in Document Interpretation
Effective AI document interpretation relies on proper training and ongoing maintenance of AI systems. Here are essential practices to enhance accuracy in this area.
Best Practices for Training AI Models
Training AI models requires a focused approach. First, data quality is crucial. Use clean, well-annotated data for training to improve the model’s ability to interpret documents correctly.
Incorporate diverse datasets to ensure the AI learns from a wide range of document types and styles. This includes technical documents, legal texts, and informal communications.
Utilizing conditional generative AI techniques can improve interpretation precision. These methods enable the model to adapt its outputs based on specific input conditions, increasing relevance.
Finally, testing the model against real-world scenarios is vital. Regular evaluations help identify weaknesses and provide insights for further training adjustments.
Maintaining AI Systems for Continued Accuracy
Ongoing maintenance is essential for sustaining AI interpretation performance. Implement routine audits of AI outputs to monitor accuracy and identify areas for improvement.
Regularly updating training datasets helps the AI adapt to changes in language and document formats. Integrating new documents reinforces its learning and keeps the model relevant.
Incorporating user feedback can also enhance performance. By leveraging insights from actual users, adjustments can be made to accommodate common interpretation challenges.
Utilizing platforms like Viggle AI allows users to refine their models with targeted data settings, enhancing interpretation capabilities. Maintaining a flexible approach to updates ensures the AI continues to meet evolving document interpretation needs.
Comments